[ad_1]
At most companies, the profile of a large-scale technology initiative has looked astonishingly similar over the last several decades. The initial pledge of US$30 million and two years soon balloons to $50 million, and two years becomes four. And this pattern of heavy investment shows no sign of slowing down: Gartner predicts that global spending on enterprise software will grow 9.5 percent in 2018, reaching $389 billion — up from $355 billion in 2017.
We can think of these traditional IT initiatives as “big” automation, with a nod to their big budgets, big timelines, and big functional agendas. Enterprise resource planning (ERP) platforms, customer relationship management software, and product life-cycle management software, among other systems, have helped companies streamline functions and track data. However, many of these automated systems still require significant manual processing. For example, PwC’s 2017 Finance Effectiveness Benchmark Report found that finance professionals spend half their time focused on the mundane, repetitive task of gathering data from various systems dependent on structured databases. As a result, although enterprise IT remains essential to companies’ business operations, many of these systems have reached the point of diminishing returns.
Fortunately, the speed, scale, and cost of automation are evolving beyond this point. Intelligent technologies such as robotic process automation, machine learning, and natural language processing systems offer companies new opportunities to improve process performance and realize significant cost savings. These technologies can be implemented in short sprints, focused on a specific problem, with manageable costs. Although big automation involves creating core enterprise systems that drive commonality, standardization, and centralized control, “small” automation is the fast implementation of flexible and adaptable technologies that fill the gaps left by your current enterprise systems — enabling new levels of productivity.
The potential impact is striking: Small automation can improve the productivity of individual processes by 80 to 100 percent (the top of the range reflects a transition to a touchless solution) and overall functions by 20 percent or more. The PwC benchmark report cited above found that in the finance function, automation could reduce by up to 46 percent the time and cost of key processes such as billing, management reporting, general accounting, and budgeting. Finance professionals then would be able to focus on higher-value activities, such as analysis that could lead to breakthrough insight. The same level of improvement is possible in other functions, such as customer service, supply chain management, and sales. This ability to economically solve problems at the team or even task level marks a significant change in business technology.
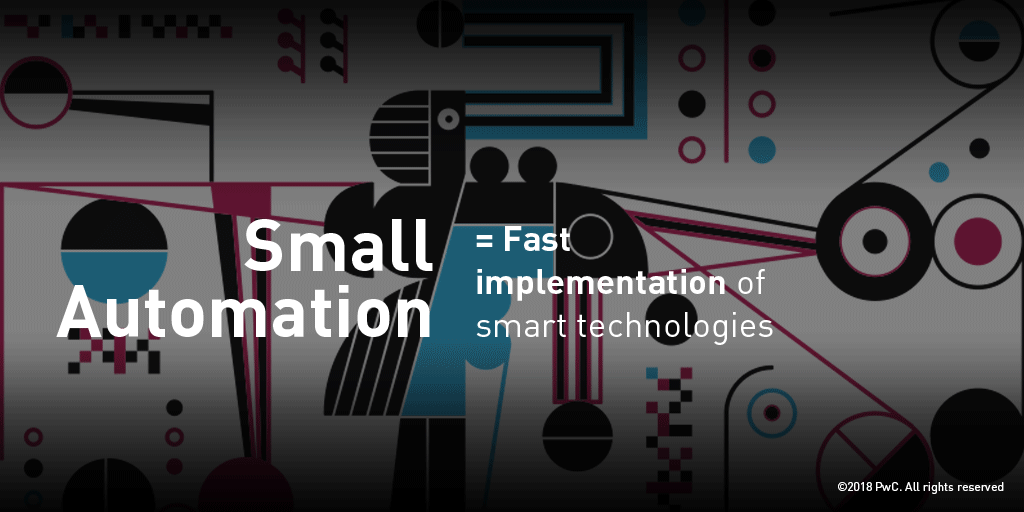
Enterprise IT Gets Flexible
Small automation does not replace big automation. Instead, it relies on new technologies to build on the foundation of data and standardized processes that large-scale IT initiatives have established. The small automation approach has several key differences from big automation that enable process improvements.
Small automation can improve the productivity of individual processes by 80 to 100 percent, and overall functions by 20 percent or more.
For one, small automation is easier to implement and much less expensive: A sprint can come with a price tag as low as tens of thousands of dollars, versus the tens of millions typically spent on big automation efforts. And large-scale IT initiatives usually apply to an entire function, such as finance or sales. This involves significant coordination among the various functional leaders and frontline employees. But small automation can be applied to individual processes or tasks. For example, a company could put a bot in place to pull specific finance data from a spreadsheet and load it to the company’s ERP platform. Because such tools can be so targeted, they do not require institutional buy-in.
Small automation is also far more flexible than big automation. Traditionally, companies could not begin to capture gains from automation technology until they had standardized processes and databases that were accessible to a broad set of users, which could take years. Before the process itself could be implemented, committees had to agree on what the optimal processes were so employees at different sites would all execute that process the same way. Small automation, in contrast, does not depend on standardization. For example, intelligent process automation platform software such as machine learning can accommodate differences in terminology and other types of complexity, offering immediate gains. This software can also bring together multiple data sets in the cloud and allow for the use of more unstructured data.
Finally, the technologies enabling small automation are easily configurable and able to learn dynamically, rather than relying on predefined rules or programmers to write the perfect algorithm in advance. This allows companies to deploy small automation in environments where the input and output are highly variable, such as service centers that manage multiple forms of inquiry and require tailored responses. New automation technologies work with those variations, rather than requiring someone to manually process and standardize the data beforehand. For instance, a chatbot can learn about a company’s benefits plan — its various policies and protocols — and instantly provide answers to questions specific to an individual employee’s package.
Employees will be used to large-scale IT projects that overpromise and under-deliver. Demonstrating strong returns in a short time will convert the cynics.
Consider a company with multiple units and departments that buy products and services from different vendors. Management wants to bundle those purchases to reduce complexity and become more efficient, but because the purchases are made on different systems, the company has limited transparency regarding who’s buying what (or what they’re paying). The traditional, big automation solution: Standardize processes, data, and systems across the entire enterprise — a time-consuming and expensive undertaking.
Now contrast this scenario with a small automation approach. With a properly trained algorithm and a sufficiently sized historical data set, the company uses machine learning to identify patterns in the purchasing data — even when the data has inconsistencies — reducing redundant purchases and streamlining the entire process.
A machine could be tasked with sifting through historical data to determine which vendors for a particular service offer the best value: the best price, quality, delivery or financing terms, or some other metric. But the underlying information does not need to be standardized or even labeled consistently. One business unit might have categorized a vendor as providing “secretarial services,” another might have it as “administrative support,” and a third might call it “clerical contracting.” Machines are smart enough to scan the data and derive a synthetic master data model to see that those three terms all refer to the same service, group them together, and assess them accordingly.
Using existing data, in whatever form, machines also can spot historical patterns, determine how vendors have performed, recommend one vendor, and even make predictions about future transactions (for example, the potential volume discount a company can demand by bundling purchases for different units). And critically, all of this happens instantly: Buyers can direct their orders to the preferred supplier in real time, or the system can do that for them automatically. This wasn’t possible before, when the information was fractured across multiple ERPs.
The Small Automation Mind-Set
The distinctions between small automation and big automation offer companies a fundamentally different level of operational performance and cost. But adopting the small automation approach can be challenging at organizations where large-scale initiatives have long been the norm, because it requires a significant change in mind-set. Company leaders should start with the following three steps.
1. Focus on value. Because small automation can solve targeted problems, it’s up to company leaders to identify applications that offer the most potential value. Leaders must stay true to the promise of small automation and avoid getting overly ambitious in scope — for example, by aiming for the large-scale consolidation or standardization that employees have grown accustomed to. Employees will be used to projects that overpromise and under-deliver. Demonstrating strong returns in a short time will convert the cynics. Small automation projects typically happen in a series of sprints, and can be completed in 16 to 20 weeks.
2. Consider the future of work. Automation alone does not save money or improve performance: People and processes will also have to change. This is an area where there is understandable anxiety; automation stokes fears that machines will take people’s jobs. But our experience suggests that many of the tasks being automated are activities people tend not to want to undertake, such as spending half the day pulling and loading data. Or they are tasks for which small automation can actually improve people’s performance — for instance, by introducing predictive algorithms that help them make better decisions and free up their time for more rewarding, interesting, and higher-value-add activities.
This is an entirely new way of working, and company leaders will need to ensure that both they and their people have the right knowledge and skills — such as programming and data science knowledge and process improvement skills — to be successful. And they’ll need to ensure that everyone’s mind-set and behaviors also shift accordingly. People will need to be able to accurately align automation opportunities with fit-for-purpose automation types (big or small), accelerate time-to-value from automation by breaking solutions into minimum viable products, and incorporate predictive algorithms into real-time workflows to drive better decision making.
3. Take a big-picture view. The backbone of this new way of working is strategy. Companies need to know their own strengths. There will be “keep the lights on” activities that can become touchless, as well as differentiating activities that should become increasingly intelligent. Moreover, companies need to take a fresh look at their organizational structure to ensure that it gives teams the freedom to develop creative solutions and experiment. Small automation works well at the level of individual processes or tasks. To that end, the company should put some guardrails in place to ensure accountability, and then let business units and departments adopt the tools as they see fit.
Large-scale systems, though challenging to implement, have created troves of valuable data in nearly every corporate business unit and function. Now, with new technologies at the ready, companies can make this data work for them in ways that were mostly unheard of only two or three years ago. The next generation of automation is small.
Author Profiles:
- Dan Priest is a thought leader in digital and technology strategy for Strategy&, PwC’s strategy consulting business. Based in Los Angeles, he is a principal with PwC US and is responsible for developing and executing digital strategies with clients across industries.
- Kumar Krishnamurthy is a leading practitioner in IT strategies for Strategy&. Based in Chicago, he is a principal with PwC US. He works with global enterprises on technology strategy and operating model design, and enabling a productivity agenda leveraging automation.
- Alex Blanter is a principal with PwC US, based in Silicon Valley. He is a leader in the firm’s product and operations team in the telecommunications, media, and telecom industry, working with companies on using emerging technologies to drive business value.
[ad_2]
Source link
Leave a Reply